Right down to a cellular level, life requires water for survival.
Predicting how liquid H2O squeezes its way through molecular-scale plumbing requires a level of simulation that currently taxes even the most powerful computers.
So researchers in the US turned to machine learning to figure out how water’s electric properties change when trapped inside nanometer-sized cylinders made of pure carbon.
Don’t let water’s apparent simplicity fool you. Inside every molecule there’s a single oxygen bullying its hydrogen sidekicks for more than its fair share of electron time, creating an imbalance in charge referred to as a dipole.
This imbalance gives water its mix of unusual properties, allowing it to loosely stick together in ways that account for surface tension, or to spread out in a wide variety of formations as it freezes into ice.
Packed inside a hydrophobic carbon nanotube that a garden variety virus might regard as the perfect size for a coffee mug, water molecules boost interactions with an electric field thanks to their confinement.
Knowing exactly how and why this occurs hasn’t been fully described.
“It’s necessary to understand the ability of the confined liquid to screen electric fields and how this varies from the bulk environment,” says lead author Marcos Calegari Andrade, a materials scientist at Lawrence Livermore National Laboratory (LLNL).
“An improved understanding of the dielectric response of confined water is important not only for advancing separation technologies but also for other emerging applications, such as energy storage and conversion.”
The dielectric effect describes how materials such as water respond to electric fields. Where conducting materials like copper wires transfer electric charges in the form of a current, the charged components of dielectric materials swivel to align themselves in ways that feed back into the broader electric field.
Packing water molecules into carbon nanotubes narrower than 10 nanometers in diameter has revealed new phases of water in the past and been shown to facilitate a far more rapid transfer of protons down one-dimensional chains of water molecules.
Expanding the pore size has also hinted at the formation of ice structures that wouldn’t be seen in larger bodies of water.
Applying a theoretical framework to explain these experimental results is easier said than done, however. It’s possible to build a relatively complete picture of molecular behavior by building simulations up from first principles, but only for a few hundred atoms over timescales of a tiny fraction of a second.
To determine dielectric constants running in other directions through the chamber, researchers ran fundamental principles through a machine learning process. This allowed for a more complete picture that included quantum effects to calculate potential energy and describe the wibble-wobble of individual molecules.
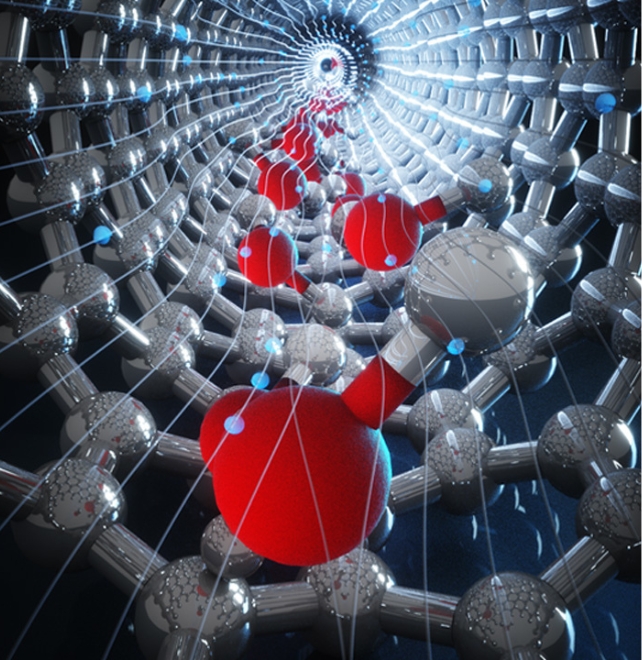
Their approach revealed an electronic structure that wouldn’t have been apparent under conventional simulations, one that builds parallel to the tube’s walls running down the axis of the water column.
In their simulation, the dielectric constant on the axis of the carbon nanotubes increased as the diameter of the tubes got smaller. It peaked at 0.79 nanometers, where the water molecules are forced to line up in a single file.
Mapping the enhancement of the water’s dielectric effect at these small scales could provide molecular biologists with vital clues on the flow of water and other materials through tiny cellular channels, or help researchers tailor pharmaceuticals that might operate more effectively in solutions confined in small spaces.
“Fundamental studies of the confinement effect on water’s dielectric constant are beneficial to understand and improve current technologies,” says Anh Pham, a computational materials scientist at LLNL.
This research was published in The Journal of Physical Chemistry Letters.